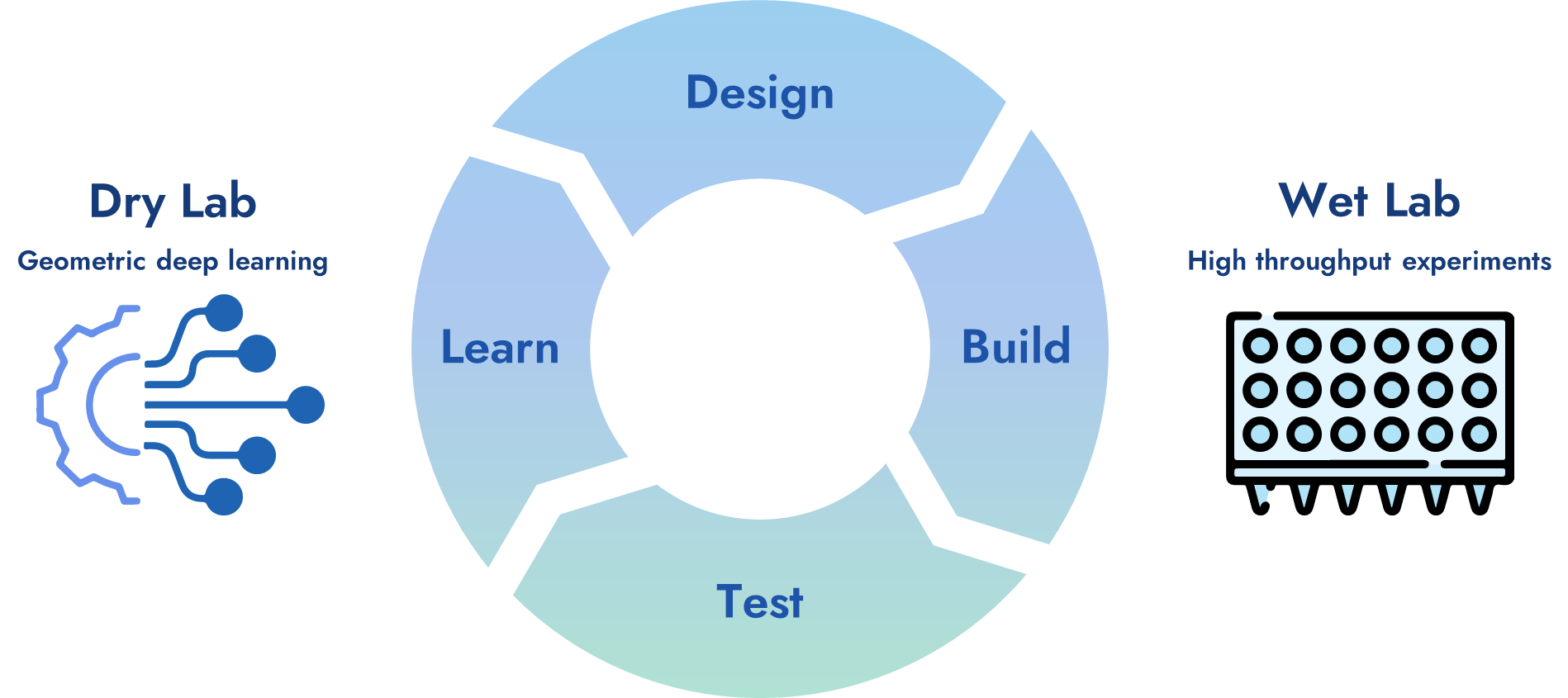
我们将AI与湿实验相结合,有效减少实验量、缩短研发周期、降低成本,在干湿循环中增强AI模型的精度和置信度,提高药物临床成功率。
生成式AI技术:迈向蛋白质从头设计
我们率先将扩散生成模型引入分子设计,设计良好的深度生成模型能够逼真地模拟蛋白质高维分布,实现高效评估样本可靠性、大批量生成新样本。
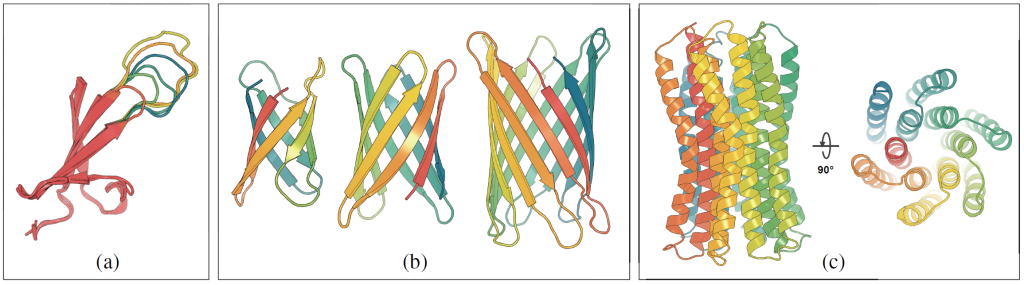
我们的优势:
- 扩大搜索空间:传统理性设计只能同时优化数个氨基酸位点,生成式AI可对整段蛋白(如HCDR3区)进行设计和优化
- 定制化生成:根据结合靶点/催化功能/性质要求设计特定的蛋白质,想象力所及,我们都努力为您实现
- 快速更新迭代:在高通量湿实验中快速迭代模型和分子,用最快速度得到最好的分子
几何深度学习:基于结构理解蛋白质
几何深度学习是一种建模分子三维结构和功能的前沿深度学习技术,将蛋白等分子在三维操作(如平移、旋转和反演)中表现出的对称性纳入了模型设计,从而大大提高了建模的准确性和效率。百奥几何已将多种自研的几何深度学习算法融入管线中。
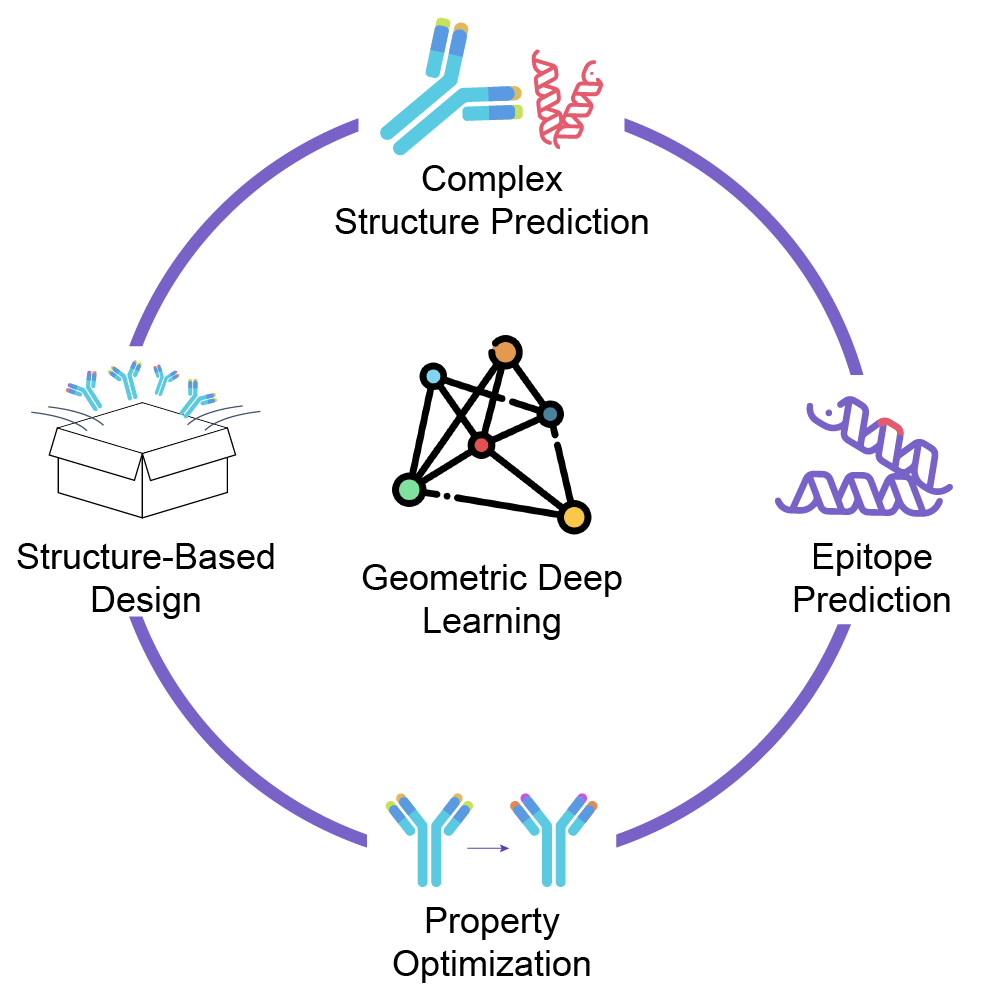
我们的优势:
- 快速精准的结构预测:自研抗体结构预测算法,比传统方法提速20倍且精度更高、误差更小
- 洞悉分子的结合模式:对结合界面的精准建模使我们能进行有效的大分子从头设计和定向进化
- 预知风险并改造分子:基于结构可预测分子稳定性、免疫原性等多种风险,结合生成式AI进行分子改造,可大大提高项目成功率
学术成果
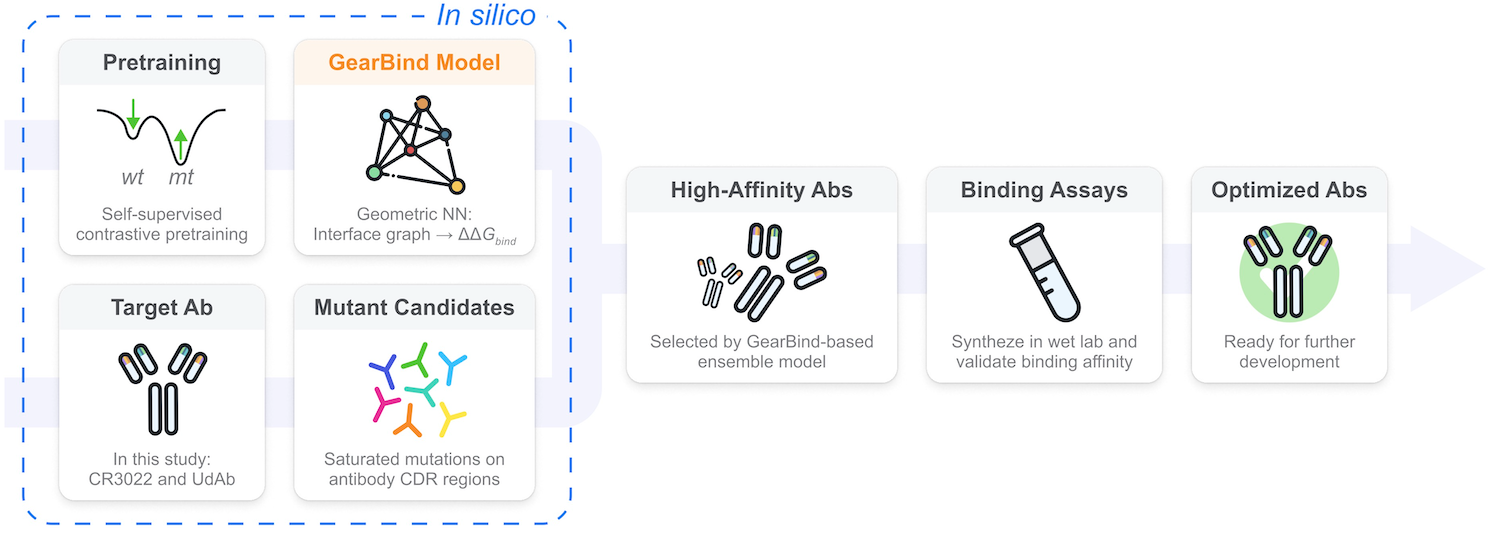
GearBind: Pretrainable geometric graph neural network for antibody affinity maturation
Geometric deep learning + contrastive pretraining yields 6x affinity in 20 samples.
Nature Communications, 2024
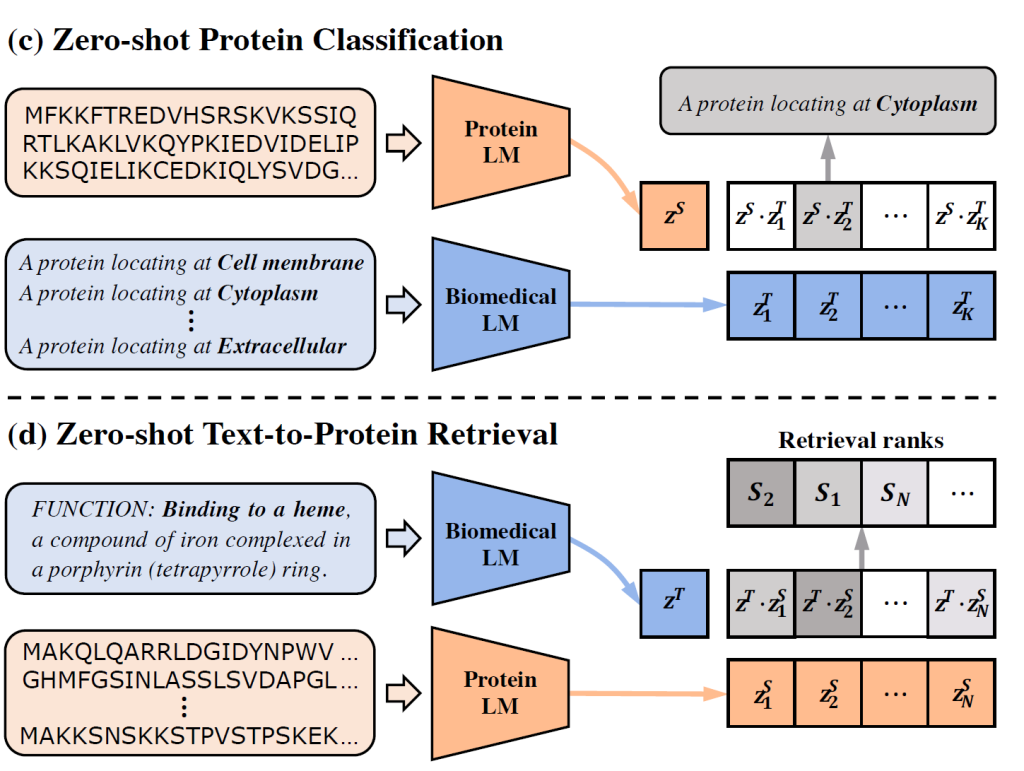
ProtST: Multi-Modality Learning of Protein Sequences and Biomedical Texts
Multimodal pretraining enables zero-shot function prediction and retrieval.
ICML 2023 Oral Presentation
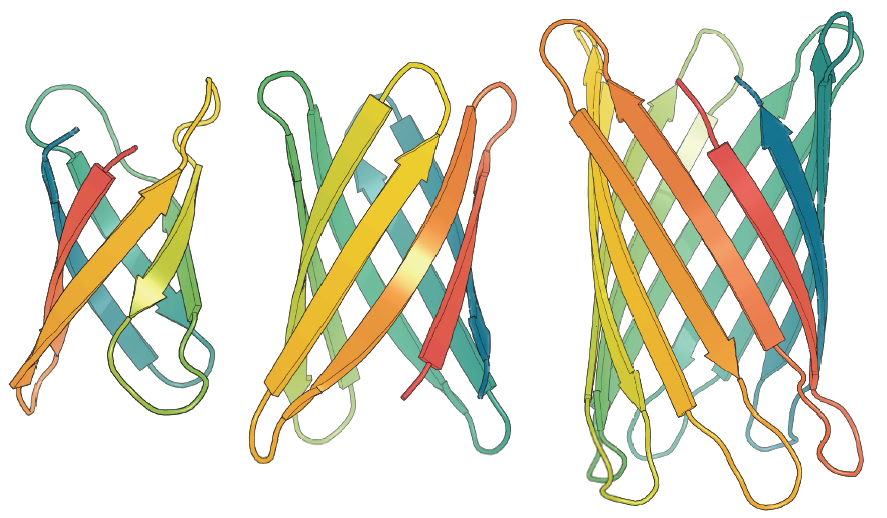
ProtSeed: Protein Sequence and Structure Co-Design with Equivariant Translation
Joint sequence-structure translation enables fast generative protein design.
ICLR 2023
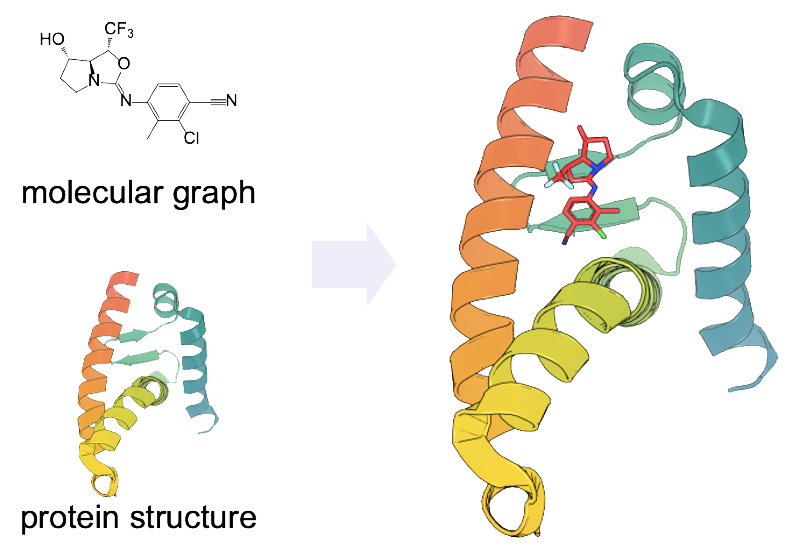
E3Bind: An End-to-End Equivariant Network for Protein-Ligand Docking
End-to-end protein-ligand docking with SE(3)-equivariance.
ICLR 2023
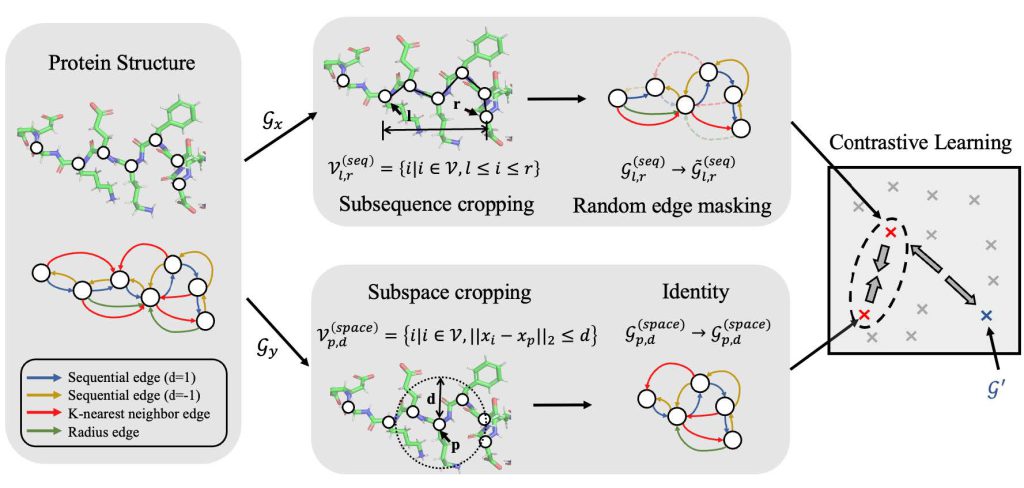
GearNet: Protein Representation Learning by Geometric Structure Pretraining
Multiview contrastive pretraining yields rich protein structure representations.
ICLR 2023
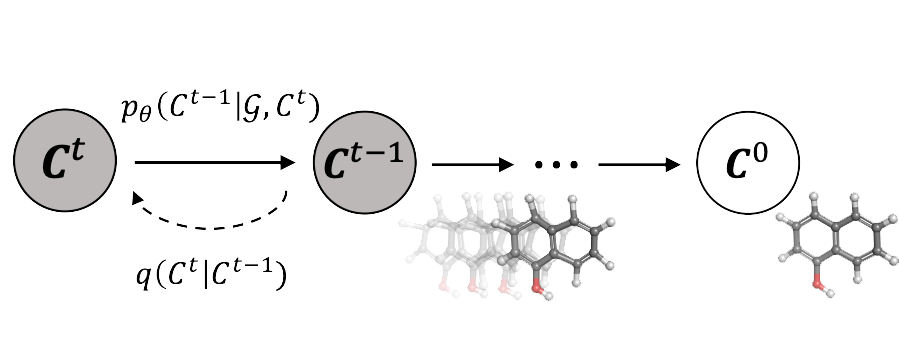
GeoDiff: A Geometric Diffusion Model for Molecular Conformation Generation
Geometric probabilistic models; Markov chains; SE(3)-equivariance; denoising diffusion.
ICLR 2022 Oral Presentation
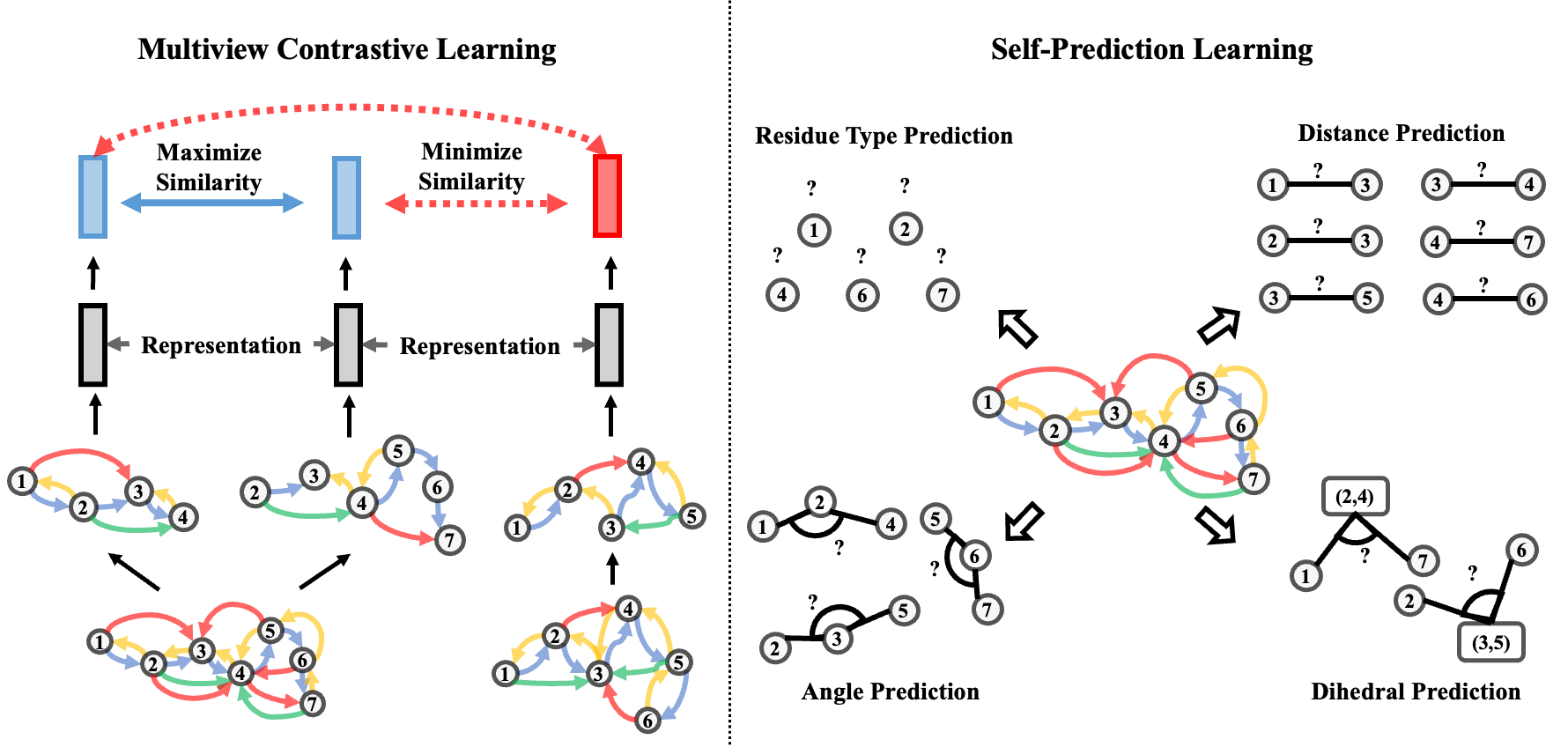
PEER: A Comprehensive and Multi-Task Benchmark for Protein Sequence Understanding
Simple, effective structure-based protein encoder coupled with geometric self-supervised learning.
NeurIPS 2022 Datasets and Benchmarks Track
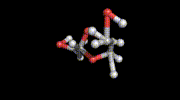
ConfGF: Learning Gradient Fields for Molecular Conformation Generation
Molecular 3D conformation generation; denoising score matching; SE(3)-equivariance.
ICML 2021 Long Talk
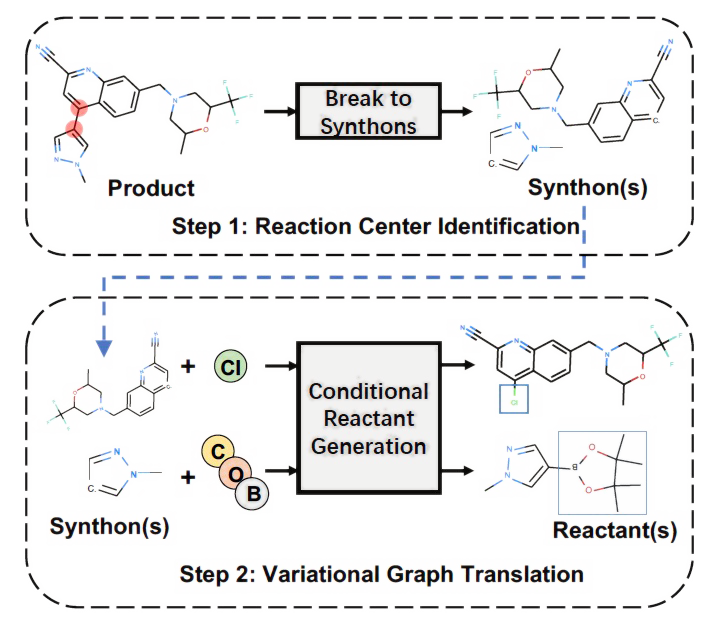
G2G: A Graph to Graphs Framework for Retrosynthesis Prediction
Retrosynthesis via graph translation; inspired by the disconnection approach in Organic Synthesis.
ICML 2020