On September 6, BioGeometry, together with the Mila Laboratory team led by Jian Tang in Canada and the teams of Tinglei Ying and Yanling Wu from Fudan University, published a paper in Nature Communications. They introduced GearBind, a pre-trained geometric graph neural network, and its application in improving antibody affinity. The results showed that GearBind not only excelled in standard datasets and independent validation sets but also successfully enhanced the affinity of two different antibodies using AI, significantly reducing the need for labor-intensive wet lab experiments. This method could save up to 70% of time and 75% of costs, marking a new era in protein-protein affinity optimization.
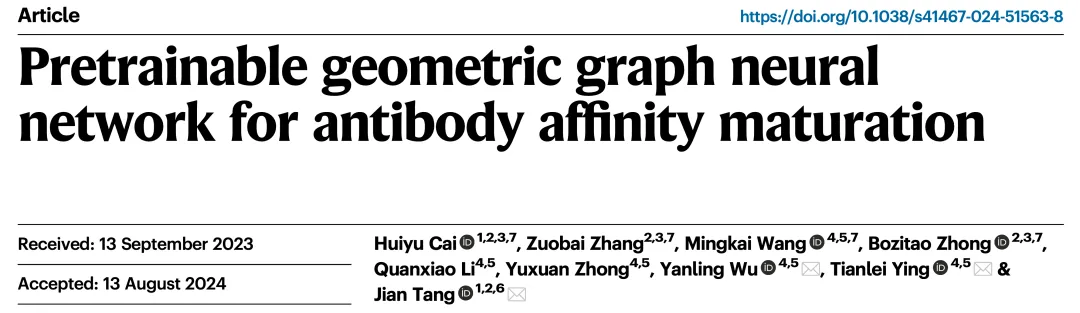
Challenges in Protein Affinity Optimization
Protein affinity optimization involves modifying protein ligands to enhance their binding to target receptors, which is crucial for developing protein-based drugs. Traditional methods rely on identifying key amino acids and screening mutants. However, even single mutations in a monoclonal antibody’s 60 CDR regions can produce over a thousand variants, and triple mutations can generate up to 230 million variants. Despite high-throughput methods, the time, cost, and labor involved in wet lab synthesis and validation remain high. Thus, computational methods to explore mutation space, identify effective mutations, and reduce wet lab workload are essential.
AI-Driven Antibody Optimization
BioGeometry’s approach to solving affinity optimization includes three main strategies:
- Using the GeoFlow model to predict the structures of protein-protein complexes that have not been experimentally determined. GeoFlow, developed by BioGeometry, has a 95% higher success rate in antigen-antibody prediction tasks compared to AlphaFold2, achieving world-class results, especially with known epitopes.
- Using the GearBind model to quickly and accurately screen ligand mutants and validate their expression. Pre-trained with extensive protein structure data, GearBind can quickly learn from a small number of wet lab results, significantly improving its performance within 1-2 rounds.
- Offering these capabilities through the AI-driven protein design and optimization platform GeoBiologics, providing users with a convenient and user-friendly experience.
This AI-driven process reduces wet lab workload through effective computations, potentially speeding up project timelines and improving outcomes. The paper details the technical implementation and applications of the first version of GearBind.
GearBind: Innovations in Algorithm Design
The GearBind algorithm, developed by the BioGeometry-Mila team, introduces three key innovations:
- Constructing a multi-relational graph among all heavy atoms at the binding interface, capturing both sequence and structural proximity.
- Using multi-level message-passing to model protein-protein interactions at the atomic, atomic pair, and amino acid levels, crucial for side-chain interactions.
- Employing contrastive learning techniques to pre-train on extensive unsupervised protein structure data, enhancing performance and understanding of side-chain interactions.
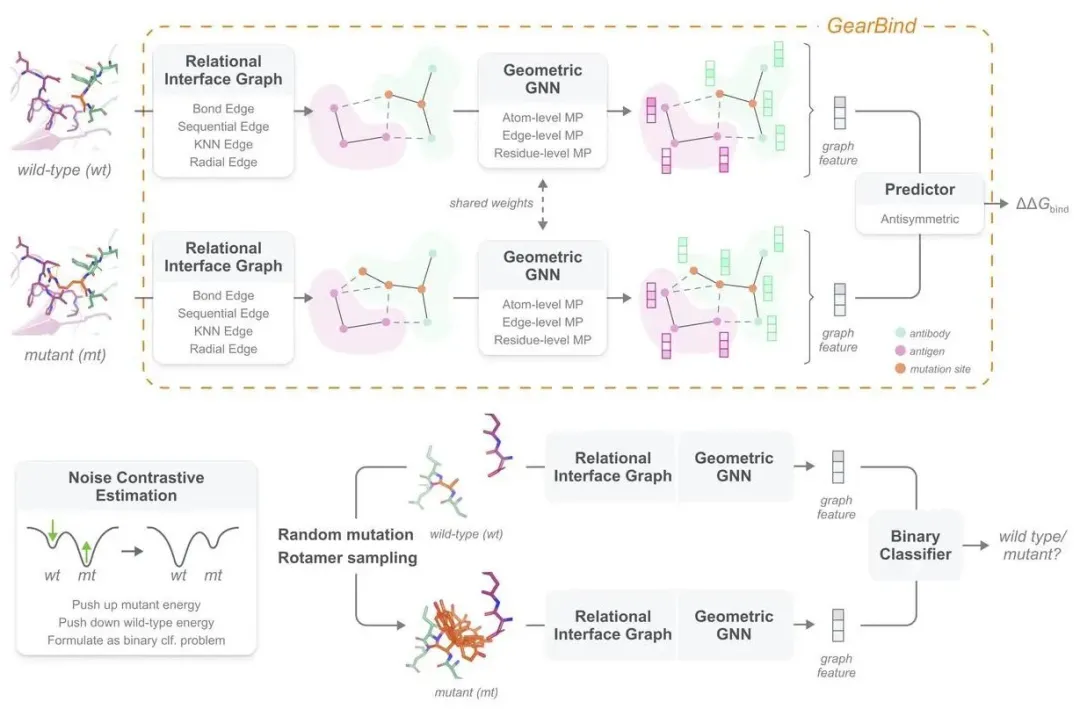
These innovations enable GearBind to outperform existing models across all metrics, with significant performance gains post pre-training.
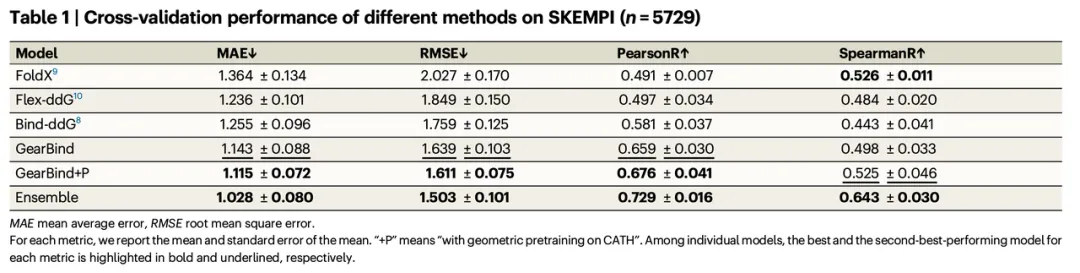
AI in Antibody Affinity Maturation
To validate the method, BioGeometry and Fudan University used AI to enhance the affinity of two antibodies with different antigens.
For the CR3022 antibody, which had reduced affinity for the Omicron variant, 9 out of 12 mutations recommended by the model improved affinity. Combining the best mutations, the second-round variants reduced EC50 for Delta RBD by 1.8-3.4 times and for Omicron Spike by 7.6-17.0 times. Overall, the team achieved up to a 17-fold reduction in EC50 for Omicron Spike and a 6.1-fold increase in affinity, reaching low nM levels.
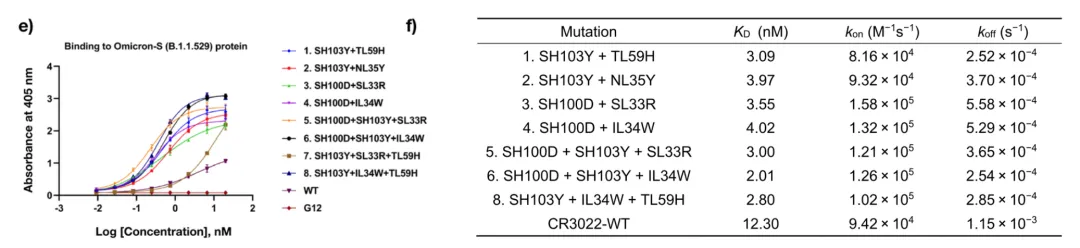
In another project, for the nanobody UdAb against the oncofetal antigen 5T4, 2 out of 12 mutations improved affinity, reducing EC50 by up to 5.6 times. Notably, the antigen epitopes for these projects were not in the training set, demonstrating the model’s strong generalization ability. After a year of iteration, BioGeometry’s affinity maturation process has been further upgraded. The new model has expanded training data and can continuously learn from wet lab data, offering stronger and more efficient performance. In a recent project, the model quickly evolved after one round, generating highly active combination mutations, increasing antibody neutralizing activity by 1000 times.
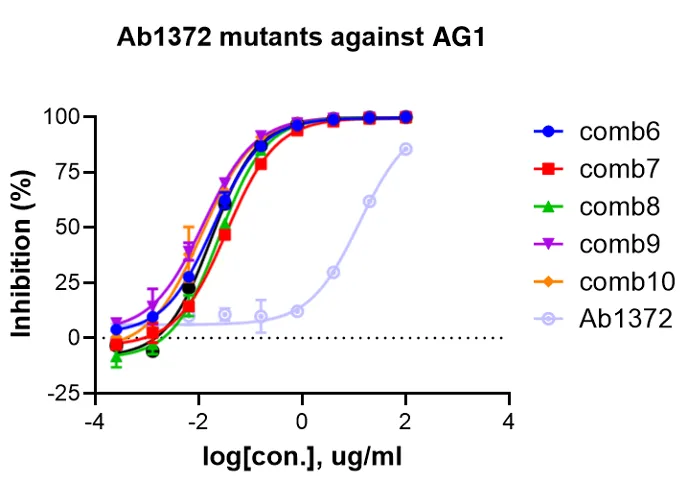
Using GearBind for Affinity Maturation
The upgraded GearBind model is now available on the GeoBiologics platform, offering AI-driven protein-protein affinity maturation services to dozens of customers. Users can upload the complex structure (or predict it using GeoFlow on the platform), select mutation sites, and quickly complete saturation mutation scans and visualization, obtaining better molecules faster.
The GeoBiologics platform also features a highly accurate structure prediction module based on GeoFlow, a one-stop macromolecule design, and multi-objective optimization module. Visit https://geobiologics.biogeom.com for more details and to apply for a trial.