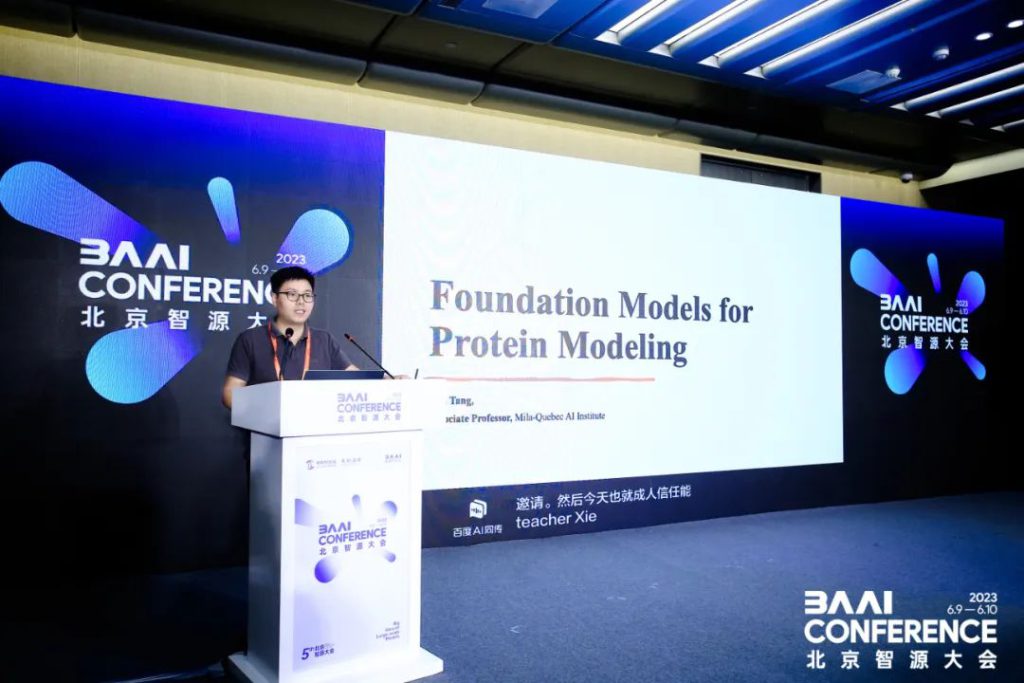
The AI Life Sciences Forum of the 2023 BAAI Conference was successfully convened in June, with the proceedings admirably hosted by Xiaoliang Sunney Xie (谢晓亮), Peking University Professor and member of the US National Academy of Sciences and the National Academy of Medicine, and co-chaired by Qiwei Ye (叶启威), head of the Health and Bio Computing Research Center, BAAI.
The forum started with a keynote speech by Nobel laureate in Chemistry, Arieh Warshel, introducing how to use AI to perform multi-scale studies in enzymes. This was followed by keynote speeches from Professor Yiqin Gao (高毅勤) of the Peking University School of Chemistry and Molecular Engineering, Associate Professor Jian Tang (唐建) of Mila – Quebec AI Institute, Tian Xu (许田), a genetics professor and vice president at Westlake University, and Ge Gao (高歌), a researcher at Peking University’s Biomedical Pioneering Innovation Center (BIOPIC). The speakers provided profound insights in their talks and discussions, highlighting the important role of AI in the field of life sciences.
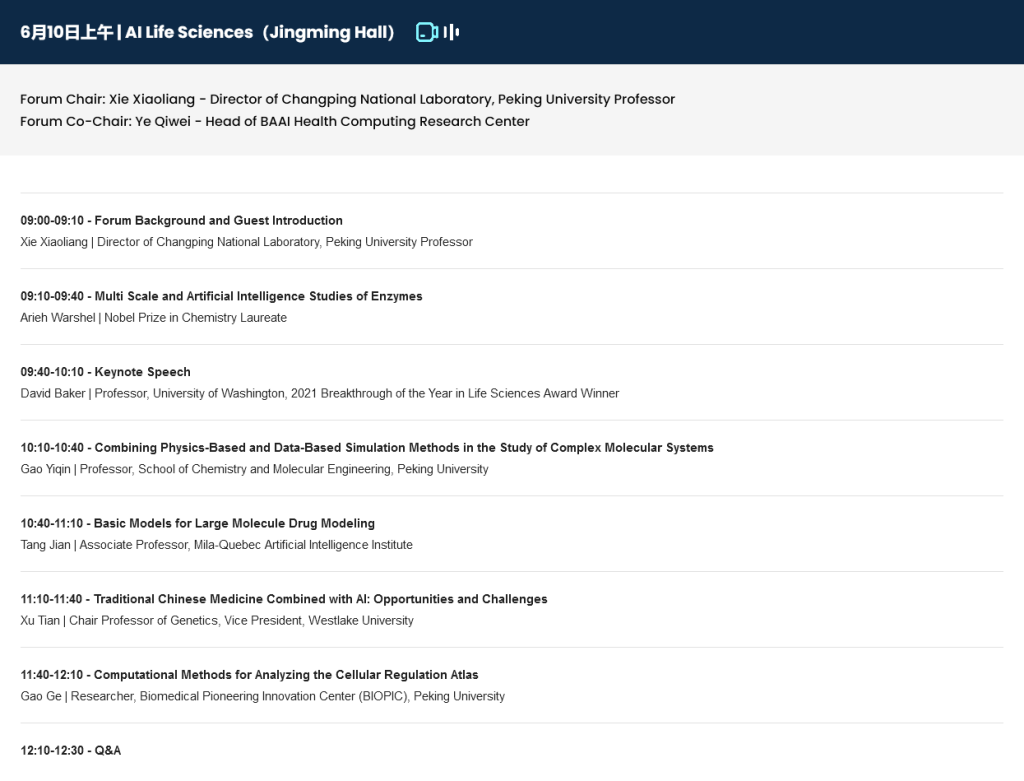
In Jian’s keynote titled “Foundation Models for Protein Modeling”, he introduced how to use graph neural networks and geometric deep learning to perform representation learning on protein structures. The GearNet method they devised not only passes information between nodes but between edges as well, making better use of the three-dimensional spatial structure of proteins. In addition, Jian proposed that multimodal learning combining protein sequence, structure and text descriptions could help us understand protein structure and function better.
On protein structure prediction, while current methods are parameter-heavy and focus on the backbone structure, Jian’s group have designed a lightweight side-chain structure prediction model that significantly improves accuracy and efficiency upon previous state-of-the-art. On protein design, he proposed a diffusion-based method that refines the structure and sequences until it gradually converges to a stable state. Inputs for this design method include the target structure and, optionally, the starting protein sequence and structure.
Finally, Jian concluded that traditional methods mainly focus on learning from protein sequence representations, but the future trend is more towards more fine-grained, structure-based representations. For example, future research could involve modeling all atoms (backbone + side-chain) in a protein system instead of using a residue-level representation. Modeling the interactions between biomolecules, i.e. the “complexes”, will be increasingly important. The ultimate goal is to design protein sequences that function better than natural proteins.